Deep Learning for Disaster Recovery
Automatic Detection of Flooded Roads
Jack Kwok is a Software Engineer with 15 years of professional experience. At Insight, he built a Deep Learning solution to automatically detect flooded roads during natural disasters. He is now a Software Engineer at Lyft working with Machine Learning and Deep Learning.
Want to learn applied Artificial Intelligence from top professionals in Silicon Valley or New York? Learn more about the Artificial Intelligenceprogram.
Flooded roads are a huge risk
With global climate change, devastating hurricanes are occurring with higher frequency.
After a hurricane, roads are often flooded or washed out, making them treacherous for motorists. According to The Weather Channel, almost two of every three U.S. flash flood deaths from 1995–2010, excluding fatalities from Hurricane Katrina, occurred in vehicles.
During my Insight A.I. Fellowship, I designed a system that detects flooded roads and created an interactive map app. Using state of the art computer vision deep learning methods, the system automatically annotates flooded, washed out, or otherwise severely damaged roads from satellite imagery.
Using Deep Learning to detect danger
Most modern machine learning techniques require labeled data. Manually labelling damaged roads on thousands of satellite map tiles would be time consuming and costly. Instead, I trained a robust, highly accurate road segmentation model from readily available satellite and street mapping data of roads in good conditions.
Then I used my model to compare road segmentation on pre-flood satellite imagery against post-flood satellite imagery to detect road changes or anomalies. With an accurate road segmentation algorithm, the difference between the post-flood segmentation and pre-flood segmentation can make a good detector for flooded roads.
The final algorithm works in 4 simple steps, outlined below.
Algorithm Overview:
- Generate road segmentation mask PreM from pre-hurricane satellite image tile.
- Generate road segmentation mask PostM from post-hurricane satellite image tile over the same area.
- Generate difference DiffM by subtracting PostM from PreM.
- Filter DiffM with ground truth street mask (derived from MapBox street map) to remove model prediction noise.
Pre-Flood (PreM)
Post-Flood (PostM)
Finally, to generate the annotated tile overlay, the non-zero-valued pixels from the last step are shaded with opaque red while the zero-valued pixels are made fully transparent so the layer can be rendered on top of any base map for presentation.
Flooded Roads(DiffM)
Choosing relevant training data
To train the road segmentation model, high quality satellite imagery and street maps from MapBox were used. Geographic locations were chosen to represent a diverse mix of street layouts, road types, and street density across the United States. The training data set consists of satellite and street map tile pairs from Boston, New York City, Atlantic City, Tampa, Miami, New Orleans, and L.A.
I chose to exclude the Houston area from the training set to ensure the final segmentation model has no knowledge about Houston. We will eventually be testing the model on the Houston area affected by Hurricane Harvey, which will provide evidence for model generalizability.
A total of 9,000 satellite tile and street mask tile pairs were used in the training set. Each map tile has dimension 512x512.
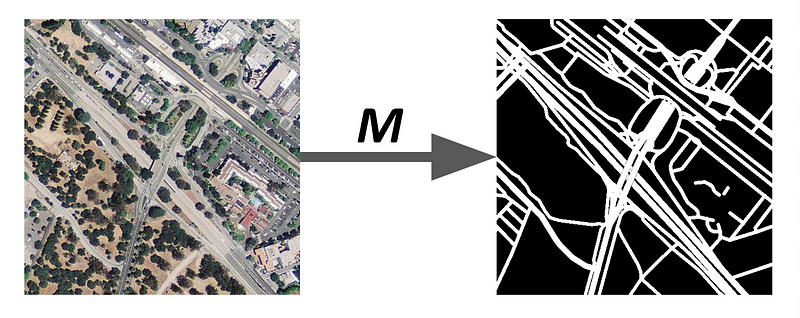
After randomization, I split the full data set into train set and validation set following a 80:20 split. I use early stopping to make sure my model does not overfit to the training data (i.e. training stops when the validation loss stops decreasing).
Picking the right model
As with image classification, convolutional neural networks (CNN) have had enormous success on image segmentation. For this project we experimented with variations of a state-of-the-art CNN model called the U-Net, originally invented for biomedical image segmentation.
The input to the U-Net is the 3 channel (RGB) satellite image array of size 512x512x3. I decided against downsampling the input images for two reasons: 1. Narrow roads become harder to detect with downsampling. 2. I aimed to train a single robust model that is able to take full advantage of high resolution satellite imagery but still can perform reasonably well on low resolution imagery. The model output is the binary segmentation mask of size 512x512. A pixel value of 1 indicates the pixel belongs to a road whereas a value of 0 indicates it does not belong to a road.
Since the network is essentially performing binary classification (road pixels vs non-road pixels) for each pixel of the input image, sigmoid activation is used as the final output layer of the U-Net.
I chose the cost function based on the negative of the dice coefficient:
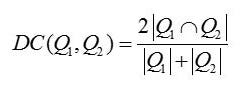
The smooth variable prevents division by zero.
Adam, the model optimizer, was initialized with a default learning rate (0.0001) and the optimizer automatically reduces learning rate by a factor of ten when the cost function for the validation set fails to decrease for two consecutive epochs. Training stops when no improvement is observed over 3 epochs.
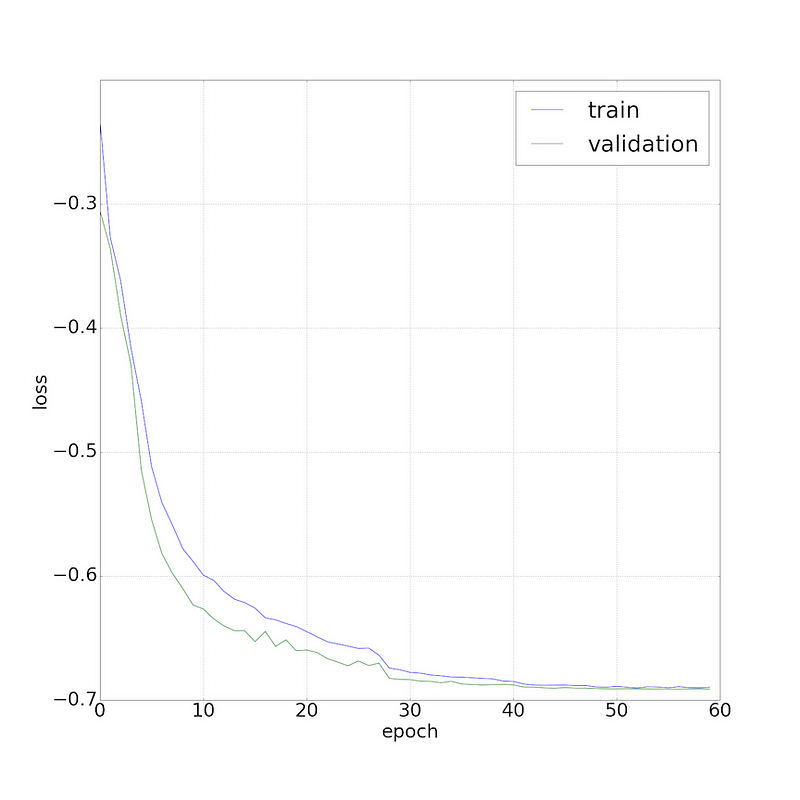
After training different variants, I eventually settled on U-Net with dilated convolutions. The benefit of dilated convolution is it increases the receptive field so that large contextual features are recognized. When multiple dilated convolutional layers are stacked together, it creates an exponential expansion of receptive field. Roads typically span across a map tile so intuitively a wider receptive field should be advantageous. Empirically, dilation slightly improves the dice coefficient.
Data augmentation
While the model inference performance on the MapBox validation set is excellent (dice coefficient > 0.7), the model initially performed badly on post hurricane images. Upon visual inspection, I concluded the issue was due to difference in image quality. The training images were essentially taken under ideal atmospheric condition (cloud free and blur free) whereas post hurricane images are slightly blurry and noisy.
To address that deficiency, I applied Gaussian blur to a randomly chosen subset of the training images to simulate variance in image qualities and re-trained the model. The re-trained model performed significantly better on post hurricane imagery because it is robust against variations in image quality/resolution.
In addition, to increase the size of our training data set, each satellite/street map pair is randomly flipped and rotated at right angles to generate 7 additional variants of the image. Augmentation boosted the dice coefficient by approximate 0.03. Image augmentation is executed in batches on the CPU in parallel with model training on the GPU to optimize training time.
A summary of results can be found below. Please note that the best model on hurricane images (second to last row) does not obtain the best Dice score on the validation set, since we only have clean satellite images available for validation.
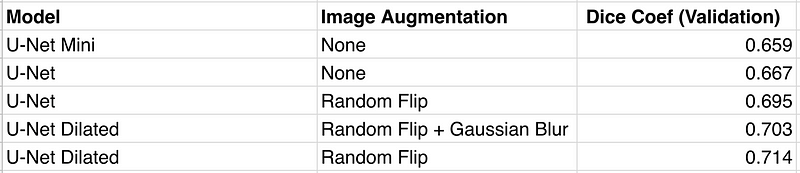
Deployment Architecture
Lastly, in order to serve the results to my website, static annotated map tiles in PNG format are published to Amazon S3 and served from CloudFront CDN to reduce network latency and increase scalability. The browser map client is built with Leaflet, an open source javascript framework for displaying maps, which supports desktop and mobile browsers.
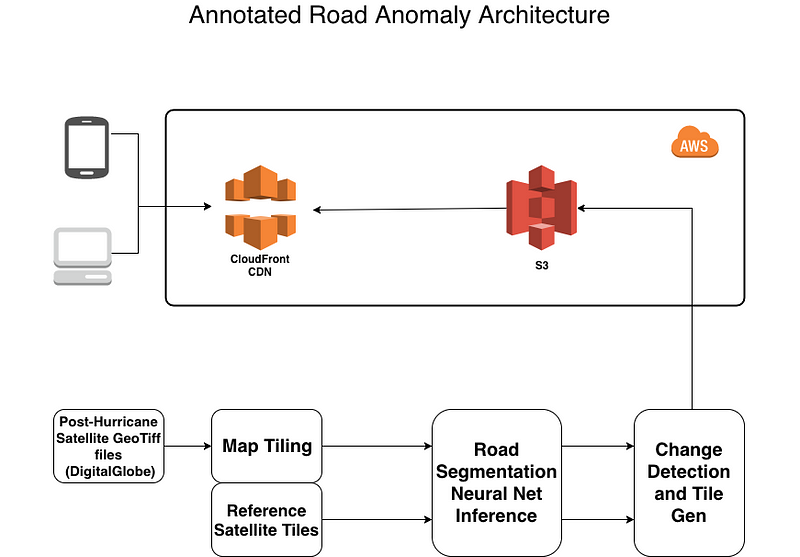
Conclusion and future directions
- The U-Net model was originally invented for biomedical image segmentation but it has proven to be highly applicable to satellite imagery.
- The accuracy of the final annotation greatly depends on the performance of the road segmentation model. My road segmentation model can be further improved by training with a more diverse dataset (particularly, images from a more diverse set of satellite sensors with different resolutions and diverse geographies).
- Due to the lack of an annotated dataset and the high cost to create one (i.e. by manually annotating flooded roads on satellite imagery using a crowdsourcing service.), I was not able to quantitatively evaluate the accuracy of the final annotation. But visual inspection shows the approach yields very good results.
Finally, the generated map annotation can be used to quickly highlight post disaster (hurricanes, landslide, earthquakes etc) road anomalies on a map. I am open sourcing this work to allow others to build upon it to make a production ready product.
Want to learn applied Artificial Intelligence from top professionals in Silicon Valley or New York? Learn more about the Artificial Intelligenceprogram.
Are you a company working in AI and would like to get involved in the Insight AI Fellows Program? Feel free to get in touch.
Deep Learning for Disaster Recovery – Insight Data
https://blog.insightdatascience.com/deep-learning-for-disaster-recov...
2017/12/15 - He is now a Software Engineer at Lyft working with Machine Learning and Deep Learning. Want to learn applied Artificial Intelligence from top professionals in Silicon ... During my Insight A.I.Fellowship, I designed a system that detects flooded roads and created an interactive map app. Using state of the art computer vision deep learning methods, the ... The smooth variable prevents division by zero. Adam, the model optimizer, was initialized with a default learning ...
https://blog.insightdatascience.com/deep-learning-for-disaster-recovery-45c8cd174d7a
とても興味深く読みました:
再生核研究所声明353(2017.2.2) ゼロ除算 記念日
2014.2.2 に 一般の方から100/0 の意味を問われていた頃、偶然に執筆中の論文原稿にそれがゼロとなっているのを発見した。直ぐに結果に驚いて友人にメールしたり、同僚に話した。それ以来、ちょうど3年、相当詳しい記録と経過が記録されている。重要なものは再生核研究所声明として英文と和文で公表されている。最初のものは
再生核研究所声明 148(2014.2.12): 100/0=0, 0/0=0 - 割り算の考えを自然に拡張すると ― 神の意志
で、最新のは
Announcement 352 (2017.2.2): On the third birthday of the division by zero z/0=0
である。
アリストテレス、ブラーマグプタ、ニュートン、オイラー、アインシュタインなどが深く関与する ゼロ除算の神秘的な永い歴史上の発見であるから、その日をゼロ除算記念日として定めて、世界史を進化させる決意の日としたい。ゼロ除算は、ユークリッド幾何学の変更といわゆるリーマン球面の無限遠点の考え方の変更を求めている。― 実際、ゼロ除算の歴史は人類の闘争の歴史と共に 人類の愚かさの象徴であるとしている。
心すべき要点を纏めて置きたい。
1) ゼロの明確な発見と算術の確立者Brahmagupta (598 - 668 ?) は 既にそこで、0/0=0 と定義していたにも関わらず、言わば創業者の深い考察を理解できず、それは間違いであるとして、1300年以上も間違いを繰り返してきた。
2) 予断と偏見、慣習、習慣、思い込み、権威に盲従する人間の精神の弱さ、愚かさを自戒したい。我々は何時もそのように囚われていて、虚像を見ていると 真智を愛する心を大事にして行きたい。絶えず、それは真かと 問うていかなければならない。
3) ピタゴラス派では 無理数の発見をしていたが、なんと、無理数の存在は自分たちの世界観に合わないからという理由で、― その発見は都合が悪いので ― 、弟子を処刑にしてしまったという。真智への愛より、面子、権力争い、勢力争い、利害が大事という人間の浅ましさの典型的な例である。
4) この辺は、2000年以上も前に、既に世の聖人、賢人が諭されてきたのに いまだ人間は生物の本能レベルを越えておらず、愚かな世界史を続けている。人間が人間として生きる意義は 真智への愛にある と言える。
5) いわば創業者の偉大な精神が正確に、上手く伝えられず、ピタゴラス派のような対応をとっているのは、本末転倒で、そのようなことが世に溢れていると警戒していきたい。本来あるべきものが逆になっていて、社会をおかしくしている。
6) ゼロ除算の発見記念日に 繰り返し、人類の愚かさを反省して、明るい世界史を切り拓いて行きたい。
以 上
追記:
The division by zero is uniquely and reasonably determined as 1/0=0/0=z/0=0 in the natural extensions of fractions. We have to change our basic ideas for our space and world:
Division by Zero z/0 = 0 in Euclidean Spaces
Hiroshi Michiwaki, Hiroshi Okumura and Saburou Saitoh
International Journal of Mathematics and Computation Vol. 28(2017); Issue 1, 2017), 1-16.
http://www.scirp.org/journal/alamt http://dx.doi.org/10.4236/alamt.2016.62007
http://www.ijapm.org/show-63-504-1.html
http://www.diogenes.bg/ijam/contents/2014-27-2/9/9.pdf
再生核研究所声明371(2017.6.27)ゼロ除算の講演― 国際会議 https://sites.google.com/site/sandrapinelas/icddea-2017 報告
https://ameblo.jp/syoshinoris/entry-12287338180.html
1/0=0、0/0=0、z/0=0
http://ameblo.jp/syoshinoris/entry-12276045402.html
1/0=0、0/0=0、z/0=0
http://ameblo.jp/syoshinoris/entry-12263708422.html
1/0=0、0/0=0、z/0=0
http://ameblo.jp/syoshinoris/entry-12272721615.html
ソクラテス・プラトン・アリストテレス その他
https://ameblo.jp/syoshinoris/entry-12328488611.html
ゼロ除算の論文リスト:
List of division by zero:
L. P. Castro and S. Saitoh, Fractional functions and their representations, Complex Anal. Oper. Theory {\bf7} (2013), no. 4, 1049-1063.
M. Kuroda, H. Michiwaki, S. Saitoh, and M. Yamane,
New meanings of the division by zero and interpretations on $100/0=0$ and on $0/0=0$, Int. J. Appl. Math. {\bf 27} (2014), no 2, pp. 191-198, DOI: 10.12732/ijam.v27i2.9.
T. Matsuura and S. Saitoh,
Matrices and division by zero z/0=0,
Advances in Linear Algebra \& Matrix Theory, 2016, 6, 51-58
Published Online June 2016 in SciRes. http://www.scirp.org/journal/alamt
\\ http://dx.doi.org/10.4236/alamt.201....
T. Matsuura and S. Saitoh,
Division by zero calculus and singular integrals. (Differential and Difference Equations with Applications. Springer Proceedings in Mathematics \& Statistics.)
T. Matsuura, H. Michiwaki and S. Saitoh,
$\log 0= \log \infty =0$ and applications. (Submitted for publication).
H. Michiwaki, S. Saitoh and M.Yamada,
Reality of the division by zero $z/0=0$. IJAPM International J. of Applied Physics and Math. 6(2015), 1--8. http://www.ijapm.org/show-63-504-1....
H. Michiwaki, H. Okumura and S. Saitoh,
Division by Zero $z/0 = 0$ in Euclidean Spaces,
International Journal of Mathematics and Computation, 28(2017); Issue 1, 2017), 1-16.
H. Okumura, S. Saitoh and T. Matsuura, Relations of $0$ and $\infty$,
Journal of Technology and Social Science (JTSS), 1(2017), 70-77.
S. Pinelas and S. Saitoh,
Division by zero calculus and differential equations. (Differential and Difference Equations with Applications. Springer Proceedings in Mathematics \& Statistics).
S. Saitoh, Generalized inversions of Hadamard and tensor products for matrices, Advances in Linear Algebra \& Matrix Theory. {\bf 4} (2014), no. 2, 87--95. http://www.scirp.org/journal/ALAMT/
S. Saitoh, A reproducing kernel theory with some general applications,
Qian,T./Rodino,L.(eds.): Mathematical Analysis, Probability and Applications - Plenary Lectures: Isaac 2015, Macau, China, Springer Proceedings in Mathematics and Statistics, {\bf 177}(2016), 151-182. (Springer) .
アインシュタインも解決できなかった「ゼロで割る」問題
http://matome.naver.jp/odai/2135710882669605901
Title page of Leonhard Euler, Vollständige Anleitung zur Algebra, Vol. 1 (edition of 1771, first published in 1770), and p. 34 from Article 83, where Euler explains why a number divided by zero gives infinity.
https://notevenpast.org/dividing-nothing/
私は数学を信じない。 アルバート・アインシュタイン / I don't believe in mathematics. Albert Einstein→ゼロ除算ができなかったからではないでしょうか。
ドキュメンタリー 2017: 神の数式 第2回 宇宙はなぜ生まれたのか
https://www.youtube.com/watch?v=iQld9cnDli4
〔NHKスペシャル〕神の数式 完全版 第3回 宇宙はなぜ始まったのか
https://www.youtube.com/watch?v=DvyAB8yTSjs&t=3318s
NHKスペシャル〕神の数式 完全版 第1回 この世は何からできているのか
https://www.youtube.com/watch?v=KjvFdzhn7Dc
NHKスペシャル 神の数式 完全版 第4回 異次元宇宙は存在するか
https://www.youtube.com/watch?v=fWVv9puoTSs
ゼロ除算の論文
Mysterious Properties of the Point at Infinity
https://arxiv.org/abs/1712.09467
Algebraic division by zero implemented as quasigeometric multiplication by infinity in real and complex multispatial hyperspaces
Author: Jakub Czajko, 92(2) (2018) 171-197
WSN 92(2) (2018) 171-197
http://www.worldscientificnews.com/wp-content/uploads/2017/12/WSN-922-2018-171-197.pdf
2014.2.2 に 一般の方から100/0 の意味を問われていた頃、偶然に執筆中の論文原稿にそれがゼロとなっているのを発見した。直ぐに結果に驚いて友人にメールしたり、同僚に話した。それ以来、ちょうど3年、相当詳しい記録と経過が記録されている。重要なものは再生核研究所声明として英文と和文で公表されている。最初のものは
再生核研究所声明 148(2014.2.12): 100/0=0, 0/0=0 - 割り算の考えを自然に拡張すると ― 神の意志
で、最新のは
Announcement 352 (2017.2.2): On the third birthday of the division by zero z/0=0
である。
アリストテレス、ブラーマグプタ、ニュートン、オイラー、アインシュタインなどが深く関与する ゼロ除算の神秘的な永い歴史上の発見であるから、その日をゼロ除算記念日として定めて、世界史を進化させる決意の日としたい。ゼロ除算は、ユークリッド幾何学の変更といわゆるリーマン球面の無限遠点の考え方の変更を求めている。― 実際、ゼロ除算の歴史は人類の闘争の歴史と共に 人類の愚かさの象徴であるとしている。
心すべき要点を纏めて置きたい。
1) ゼロの明確な発見と算術の確立者Brahmagupta (598 - 668 ?) は 既にそこで、0/0=0 と定義していたにも関わらず、言わば創業者の深い考察を理解できず、それは間違いであるとして、1300年以上も間違いを繰り返してきた。
2) 予断と偏見、慣習、習慣、思い込み、権威に盲従する人間の精神の弱さ、愚かさを自戒したい。我々は何時もそのように囚われていて、虚像を見ていると 真智を愛する心を大事にして行きたい。絶えず、それは真かと 問うていかなければならない。
3) ピタゴラス派では 無理数の発見をしていたが、なんと、無理数の存在は自分たちの世界観に合わないからという理由で、― その発見は都合が悪いので ― 、弟子を処刑にしてしまったという。真智への愛より、面子、権力争い、勢力争い、利害が大事という人間の浅ましさの典型的な例である。
4) この辺は、2000年以上も前に、既に世の聖人、賢人が諭されてきたのに いまだ人間は生物の本能レベルを越えておらず、愚かな世界史を続けている。人間が人間として生きる意義は 真智への愛にある と言える。
5) いわば創業者の偉大な精神が正確に、上手く伝えられず、ピタゴラス派のような対応をとっているのは、本末転倒で、そのようなことが世に溢れていると警戒していきたい。本来あるべきものが逆になっていて、社会をおかしくしている。
6) ゼロ除算の発見記念日に 繰り返し、人類の愚かさを反省して、明るい世界史を切り拓いて行きたい。
以 上
追記:
The division by zero is uniquely and reasonably determined as 1/0=0/0=z/0=0 in the natural extensions of fractions. We have to change our basic ideas for our space and world:
Division by Zero z/0 = 0 in Euclidean Spaces
Hiroshi Michiwaki, Hiroshi Okumura and Saburou Saitoh
International Journal of Mathematics and Computation Vol. 28(2017); Issue 1, 2017), 1-16.
http://www.scirp.org/journal/alamt http://dx.doi.org/10.4236/alamt.2016.62007
http://www.ijapm.org/show-63-504-1.html
http://www.diogenes.bg/ijam/contents/2014-27-2/9/9.pdf
再生核研究所声明371(2017.6.27)ゼロ除算の講演― 国際会議 https://sites.google.com/site/sandrapinelas/icddea-2017 報告
https://ameblo.jp/syoshinoris/entry-12287338180.html
1/0=0、0/0=0、z/0=0
http://ameblo.jp/syoshinoris/entry-12276045402.html
1/0=0、0/0=0、z/0=0
http://ameblo.jp/syoshinoris/entry-12263708422.html
1/0=0、0/0=0、z/0=0
http://ameblo.jp/syoshinoris/entry-12272721615.html
ソクラテス・プラトン・アリストテレス その他
https://ameblo.jp/syoshinoris/entry-12328488611.html
ゼロ除算の論文リスト:
List of division by zero:
L. P. Castro and S. Saitoh, Fractional functions and their representations, Complex Anal. Oper. Theory {\bf7} (2013), no. 4, 1049-1063.
M. Kuroda, H. Michiwaki, S. Saitoh, and M. Yamane,
New meanings of the division by zero and interpretations on $100/0=0$ and on $0/0=0$, Int. J. Appl. Math. {\bf 27} (2014), no 2, pp. 191-198, DOI: 10.12732/ijam.v27i2.9.
T. Matsuura and S. Saitoh,
Matrices and division by zero z/0=0,
Advances in Linear Algebra \& Matrix Theory, 2016, 6, 51-58
Published Online June 2016 in SciRes. http://www.scirp.org/journal/alamt
\\ http://dx.doi.org/10.4236/alamt.201....
T. Matsuura and S. Saitoh,
Division by zero calculus and singular integrals. (Differential and Difference Equations with Applications. Springer Proceedings in Mathematics \& Statistics.)
T. Matsuura, H. Michiwaki and S. Saitoh,
$\log 0= \log \infty =0$ and applications. (Submitted for publication).
H. Michiwaki, S. Saitoh and M.Yamada,
Reality of the division by zero $z/0=0$. IJAPM International J. of Applied Physics and Math. 6(2015), 1--8. http://www.ijapm.org/show-63-504-1....
H. Michiwaki, H. Okumura and S. Saitoh,
Division by Zero $z/0 = 0$ in Euclidean Spaces,
International Journal of Mathematics and Computation, 28(2017); Issue 1, 2017), 1-16.
H. Okumura, S. Saitoh and T. Matsuura, Relations of $0$ and $\infty$,
Journal of Technology and Social Science (JTSS), 1(2017), 70-77.
S. Pinelas and S. Saitoh,
Division by zero calculus and differential equations. (Differential and Difference Equations with Applications. Springer Proceedings in Mathematics \& Statistics).
S. Saitoh, Generalized inversions of Hadamard and tensor products for matrices, Advances in Linear Algebra \& Matrix Theory. {\bf 4} (2014), no. 2, 87--95. http://www.scirp.org/journal/ALAMT/
S. Saitoh, A reproducing kernel theory with some general applications,
Qian,T./Rodino,L.(eds.): Mathematical Analysis, Probability and Applications - Plenary Lectures: Isaac 2015, Macau, China, Springer Proceedings in Mathematics and Statistics, {\bf 177}(2016), 151-182. (Springer) .
アインシュタインも解決できなかった「ゼロで割る」問題
http://matome.naver.jp/odai/2135710882669605901
Title page of Leonhard Euler, Vollständige Anleitung zur Algebra, Vol. 1 (edition of 1771, first published in 1770), and p. 34 from Article 83, where Euler explains why a number divided by zero gives infinity.
https://notevenpast.org/dividing-nothing/
私は数学を信じない。 アルバート・アインシュタイン / I don't believe in mathematics. Albert Einstein→ゼロ除算ができなかったからではないでしょうか。
ドキュメンタリー 2017: 神の数式 第2回 宇宙はなぜ生まれたのか
https://www.youtube.com/watch?v=iQld9cnDli4
〔NHKスペシャル〕神の数式 完全版 第3回 宇宙はなぜ始まったのか
https://www.youtube.com/watch?v=DvyAB8yTSjs&t=3318s
NHKスペシャル〕神の数式 完全版 第1回 この世は何からできているのか
https://www.youtube.com/watch?v=KjvFdzhn7Dc
NHKスペシャル 神の数式 完全版 第4回 異次元宇宙は存在するか
https://www.youtube.com/watch?v=fWVv9puoTSs
ゼロ除算の論文
Mysterious Properties of the Point at Infinity
https://arxiv.org/abs/1712.09467
Algebraic division by zero implemented as quasigeometric multiplication by infinity in real and complex multispatial hyperspaces
Author: Jakub Czajko, 92(2) (2018) 171-197
WSN 92(2) (2018) 171-197
http://www.worldscientificnews.com/wp-content/uploads/2017/12/WSN-922-2018-171-197.pdf
再生核研究所声明 375 (2017.7.21):ブラックホール、ゼロ除算、宇宙論
本年はブラックホール命名50周年とされていたが、最近、wikipedia で下記のように修正されていた:
名称[編集]
"black hole"という呼び名が定着するまでは、崩壊した星を意味する"collapsar"[1](コラプサー)などと呼ばれていた。光すら脱け出せない縮退星に対して "black hole" という言葉が用いられた最も古い印刷物は、ジャーナリストのアン・ユーイング (Ann Ewing) が1964年1月18日の Science News-Letter の "'Black holes' in space" と題するアメリカ科学振興協会の会合を紹介する記事の中で用いたものである[2][3][4]。一般には、アメリカの物理学者ジョン・ホイーラーが1967年に "black hole" という名称を初めて用いたとされるが[5]、実際にはその年にニューヨークで行われた会議中で聴衆の一人が洩らした言葉をホイーラーが採用して広めたものであり[3]、またホイーラー自身は "black hole" という言葉の考案者であると主張したことはない[3]。https://ja.wikipedia.org/wiki/%E3%83%96%E3%83%A9%E3%83%83%E3%82%AF%E3%83%9B%E3%83%BC%E3%83%AB
世界は広いから、情報が混乱することは よく起きる状況がある。ブラックホールの概念と密接な関係のあるゼロ除算の発見(2014.2.2)については、歴史的な混乱が生じないようにと 詳しい経緯、解説、論文、公表過程など記録するように配慮してきた。
ゼロ除算は簡単で自明であると初期から述べてきたが、問題はそこから生じるゼロ除算算法とその応用であると述べている。しかし、その第1歩で議論は様々でゼロ除算自身についていろいろな説が存在して、ゼロ除算は現在も全体的に混乱していると言える。インターネットなどで参照出来る膨大な情報は、我々の観点では不適当なものばかりであると言える。もちろん学術界ではゼロ除算発見後3年を経過しているものの、古い固定観念に囚われていて、新しい発見は未だ認知されているとは言えない。最近国際会議でも現代数学を破壊するので、認められない等の意見が表明された(再生核研究所声明371(2017.6.27)ゼロ除算の講演― 国際会議 https://sites.google.com/site/sandrapinelas/icddea-2017 報告)。そこで、初等数学から、500件を超えるゼロ除算の証拠、効用の事実を示して、ゼロ除算は確定していること、ゼロ除算算法の重要性を主張し、基本的な世界を示している。
ゼロ除算について、膨大な歴史、文献は、ゼロ除算が神秘的なこととして、扱われ、それはアインシュタインの言葉に象徴される:
Here, we recall Albert Einstein's words on mathematics:
Blackholes are where God divided by zero.
I don't believe in mathematics.
George Gamow (1904-1968) Russian-born American nuclear physicist and cosmologist remarked that "it is well known to students of high school algebra" that division by zero is not valid; and Einstein admitted it as {\bf the biggest blunder of his life} (Gamow, G., My World Line (Viking, New York). p 44, 1970).
ところが結果は、実に簡明であった:
The division by zero is uniquely and reasonably determined as 1/0=0/0=z/0=0 in the natural extensions of fractions. We have to change our basic ideas for our space and world
しかしながら、ゼロ及びゼロ除算は、結果自体は 驚く程単純であったが、神秘的な新たな世界を覗かせ、ゼロ及びゼロ除算は一層神秘的な対象であることが顕になってきた。ゼロのいろいろな意味も分かってきた。 無限遠点における強力な飛び、ワープ現象とゼロと無限の不思議な関係である。アリストテレス、ユークリッド以来の 空間の認識を変える事件をもたらしている。 ゼロ除算の結果は、数理論ばかりではなく、世界観の変更を要求している。 端的に表現してみよう。 これは宇宙の生成、消滅の様、人生の様をも表しているようである。 点が球としてどんどん大きくなり、球面は限りなく大きくなって行く。 どこまで大きくなっていくかは、 分からない。しかしながら、ゼロ除算はあるところで突然半径はゼロになり、最初の点に帰するというのである。 ゼロから始まってゼロに帰する。 ―― それは人生の様のようではないだろうか。物心なしに始まった人生、経験や知識はどんどん広がって行くが、突然、死によって元に戻る。 人生とはそのようなものではないだろうか。 はじめも終わりも、 途中も分からない。 多くの世の現象はそのようで、 何かが始まり、 どんどん進み、そして、戻る。 例えばソロバンでは、願いましては で計算を始め、最後はご破産で願いましては、で終了する。 我々の宇宙も淀みに浮かぶ泡沫のようなもので、できては壊れ、できては壊れる現象を繰り返しているのではないだろうか。泡沫の上の小さな存在の人間は結局、何も分からず、われ思うゆえにわれあり と自己の存在を確かめる程の能力しか無い存在であると言える。 始めと終わり、過程も ようとして分からない。
ブラックホールとゼロ除算、ゼロ除算の発見とその後の数学の発展を眺めていて、そのような宇宙観、人生観がひとりでに湧いてきて、奇妙に納得のいく気持ちになっている。
以 上
再生核研究所声明377 (2017.8.3): ゼロの意味について
ゼロ除算について考察を深めているが、それは当然ゼロの意味を究めることに繋がる。 そこでゼロ自身についても触れてきた:
再生核研究所声明311(2016.07.05) ゼロ0とは何だろうか
この要旨は:
数字のゼロとは、実数体あるいは複素数体におけるゼロであり、四則演算で、加法における単位元(基準元)で、和を考える場合、何にゼロを加えても変わらない元として定義される。
座標系の導入における 位置の基準点を定めること。
複素平面では立体射影した場合における無限遠点が正しくゼロに対応する点である。
全ての直線はある意味で、原点、基準点を通ることが示されるが、これは無限遠点の影が投影されていると解釈され、原点はこの意味で2重性を有している、無限遠点と原点が重なっている現象を表している。古来、ゼロと無限の関係は何か通じていると感じられてきたが、その意味が、明らかになってきていると言える。
再生核研究所声明297(2016.05.19) 豊かなゼロ、空の世界、隠れた未知の世界
要旨は:
微分方程式のある項を落とした場合の解と落とす前の解を結び付ける具体的な方法として、ゼロ除算の解析の具体的な応用がある事が分かった。この事実は、広く世の現象として、面白い視点に気づかせたので、普遍的な現象として、生きた形で表現したい。
ある項を落とした微分方程式とは、逆に言えば、与えられた微分方程式はさらに 複雑な微分方程式において、沢山の項を落として考えられた簡略の微分方程式であると考えられる。どのくらいの項を落としたかと考えれば、限りない項が存在して、殆どがゼロとして消された微分方程式であると見なせる。この意味で、ゼロの世界は限りなく広がっていると考えられる。
このような視点で、人間にとって最も大事なことは 何だろうか。それは、個々の人間も、人類も 大きな存在の中の小さな存在であることを先ず自覚して、背後に存在する大いなる基礎、環境に畏敬の念を抱き、謙虚さを保つことではないだろうか。この視点では日本古来の神道の精神こそ、宗教の原点として大事では ないだろうか。未知なる自然に対する畏敬の念である。実際、日本でも、世界各地でも人工物を建設するとき、神事を行い、神の許しを求めてきたものである。その心は大いなる存在と人間の調和を志向する意味で人間存在の原理ではないだろうか。それはそもそも 原罪の概念そのものであると言える。
発想における最も大事なことに触れたが、表現したかった元を回想したい。― それは存在と非存在の間の微妙な有り様と非存在の認知できない限りない世界に想いを致す心情そのものであった。無数とも言える人間の想いはどこに消えて行ったのだろうか。先も分からず、由来も分からない。世の中は雲のような存在であると言える。
上記2件は、ゼロが基準を表すこと、無限や無限遠点の表現になっていること、消えて行った世界の神秘的な性質を述べている。
ここ3年、広くゼロ除算現象を探してきて発見したゼロの性質として、起こりえない現象や不可能性を広く表すことが分かった。
そもそもゼロで割る問題とは、最も簡単な方程式 ax=b をa=0の場合に考える事と解釈できる。一般的に考えられる解(一般逆)として、x=0 が得られると解釈できるが、その心として、神は混乱が起きたとき、元に、基準に戻る、最も簡単なものを選択すると理解できるが、これは、不可能性をも表現していると言える。 これは新しい視点である。b=0 の時の解 x=0はもともとの意味でのゼロであるが、b がゼロでない時の解x=0 は不可能性を表すゼロであると言える。ゼロの2面性を主張したい。不可能性を表す例はゼロ除算の発現を広く探している折に発見された新しい視点である。
無限とは、極限値の概念で捉えられるが、定まった数のようにも扱われている。数としての無限は曖昧である。
ところが、無限大は、無限遠点は ゼロで表されることが発見され、また証明も与えられた、これはゼロ除算から導かれたが、同時にゼロの新しい意味をも受け入れる必要がある。ゼロが不可能性をも表現しているという事実である。
水平方向にx軸、鉛直上方方向にy軸をとる。 原点から,角アルファで初速度v_0
で質点を投射する。最高到達点の高さ、水平到達点までの距離、最高到達点に至るまでの時間など、引力がなければ(g=0の場合を考える)、どんどん飛んでいき無限の彼方まで、これが従来の表現であるが、ゼロ除算では、最高到達点の高さ、水平到達点までの距離、最高到達点に至るまでの時間などは全てゼロで表される。
0 件のコメント:
コメントを投稿